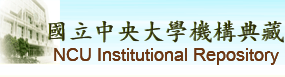 |
English
|
正體中文
|
简体中文
|
全文筆數/總筆數 : 80990/80990 (100%)
造訪人次 : 41630817
線上人數 : 3619
|
|
|
資料載入中.....
|
請使用永久網址來引用或連結此文件:
http://ir.lib.ncu.edu.tw/handle/987654321/85074
|
題名: | Anomaly Detection for PM2.5 Sensors via Transfer Learning |
作者: | 黃雪玲;Huang, Shiue-Ling |
貢獻者: | 資訊工程學系 |
關鍵詞: | 空氣品質;深度學習;異常偵測;Air quality;Deep learning;Anomaly detection |
日期: | 2021-02-23 |
上傳時間: | 2021-03-18 17:35:07 (UTC+8) |
出版者: | 國立中央大學 |
摘要: | 根據世界衛生組織估計,每年約有700萬人死於空氣汙染引發的相關疾病。在各種空氣汙染物中,PM2.5被認為是對人類影響最大的。為了監測周圍環境的PM2.5濃度,不同國家組織已經開始部署大量低成本的空氣品質感測器。然而,由於這些感測器的價格便宜,並且可能安裝在不適當的地方,因此某些空氣品質感測器的讀數可能不穩定。當使用PM2.5讀數進行數據分析時,應識別並清除這些不穩定的讀數。本文提出了一種基於深度學習的空氣品質感測器異常檢測系統。這項研究使用了兩個資料集,南海岸空氣品質管理區的PurpleAir和中央研究院的Airbox。雖然Airbox資料集中的PM2.5資料非常多,但是缺乏異常空氣品質感測器的標籤。相反,PurpleAir中氣品質感測器的分佈密度較低,但資料有室內和室外標籤。為了利用這兩個資料集,採用ADF框架標記Airbox資料集,將其用於訓練模型。然後,PurpleAir資料集用於遷移學習以重新訓練模型。PurpleAir測試集用於評估四個模型,包括來自遷移學習的LSTM模型和混合模型(將LSTM和XGBoost組合)以及僅使用PurpleAir資料集進行訓練的XGBoost和LSTM。實驗結果表明,遷移學習的過程有顯著提高了模型的性能,而且帶有遷移學習的混合模型在所有指標上均表現出最佳性能。;According to the World Health Organization, approximately 7 million people die each year from diseases caused by air pollution. Among different types of air pollutants, PM2.5 is known to be the most fatal to humans. To monitor the PM2.5 readings in the surrounding environment, several organizations in different countries have initiated to deploy a large number of low-cost air quality sensors. However, because these sensors are cheaply built and may be installed at inappropriate places, the readings of some air quality sensors may be erratic. When PM2.5 readings are used for data analysis, these erratic readings should be identified and removed. In this thesis, we propose a deep learning-based anomaly detection system for air quality sensors. The study uses two datasets, PurpleAir from South Coast Air Quality Management District and Airbox from Academia Sinica. While PM2.5 data in Airbox dataset are abundant, they lack the ground truth for anomalous air quality sensors. On the contrary, the density of air quality sensors in PurpleAir is low, but their data come with indoor and outdoor labels. To take advantage of both datasets, the ADF framework is adopted to label the Airbox dataset, which is then used to train a model. Then, the PurpleAir dataset is used for transfer learning to retrain the model. The PurpleAir test set is used to evaluate four models, including LSTM model and hybrid model (combining LSTM and XGBoost) from transfer learning and the XGBoost and LSTM that are trained using only the PurpleAir dataset. The experimental results show that the process of transfer learning significantly improves the model performance, and the hybrid model with transfer learning exhibits the best performance in all metrics. |
顯示於類別: | [資訊工程研究所] 博碩士論文
|
文件中的檔案:
檔案 |
描述 |
大小 | 格式 | 瀏覽次數 |
index.html | | 0Kb | HTML | 134 | 檢視/開啟 |
|
在NCUIR中所有的資料項目都受到原著作權保護.
|
::: Copyright National Central University. | 國立中央大學圖書館版權所有 | 收藏本站 | 設為首頁 | 最佳瀏覽畫面: 1024*768 | 建站日期:8-24-2009 :::