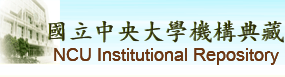 |
English
|
正體中文
|
简体中文
|
全文筆數/總筆數 : 80990/80990 (100%)
造訪人次 : 43368283
線上人數 : 1213
|
|
|
資料載入中.....
|
請使用永久網址來引用或連結此文件:
http://ir.lib.ncu.edu.tw/handle/987654321/93008
|
題名: | 基於時序卷積網路之單FMCW雷達應用於非接觸式即時生命特徵監控;Non-contact Real-Time vital sign detection Using single FMCW Radar based on Temporal Convolutional Networks |
作者: | 林以恩;Lin, Yi-En |
貢獻者: | 通訊工程學系 |
關鍵詞: | 深度學習;毫米波雷達;時序卷積網路 |
日期: | 2023-11-24 |
上傳時間: | 2024-09-19 16:38:22 (UTC+8) |
出版者: | 國立中央大學 |
摘要: | 生命特徵偵測在醫學和臨床領域中扮演著重要的角色。往年靠著接觸式裝置帶在人體上偵測並分析關鍵的生理參數,包括心率、呼吸率、心電圖等,用來協助醫生判斷病情且做出最適當的治療。在臨床診斷和監護中,生命特徵偵測的應用範圍廣泛且多樣。然而,生命特徵偵測技術也面臨著挑戰和問題。例如,穿戴裝置造成的不適感以及隱私保護等方面需要進一步的改進和研究。於是,近年開始提出非接觸式裝置檢測人體生命特徵,除了能避免長期穿戴醫療設備所帶來得不適外,比起使用 RGB 相機等設備,連續調變波雷達(Frequency modulated continuous waveform radar, FMCW)也能提供有效的隱私保護。 本篇論文的目的為非接觸式的生命特徵監控。我們提出的方法為以時 序卷積網路 (Temporal Convolutional Network, TCN) 為主體之模型用以提取生命特徵。TCN 的特點為使卷積神經網路 (Convolutional Neural Network, CNN) 也能具有時序序列建模的能力。搭配具有注意力增強功能的子系統,提供充足有效的訊息,能使模型更有效地監控生命特徵,從而提升準確度。;Detecting vital signs plays a crucial role in the medical and clinical fields. In the past, vital signs were typically measured and analyzed using contact-based devices, including heart rate monitors, respiratory rate monitors, and electrocardiography (ECG) systems, to assist doctors in diagnosing conditions and making informed treatment decisions. Vital sign detection has a wide range of applications in clinical diagnosis and monitoring. However, there are challenges and issues associated with traditional contact-based methods, such as discomfort caused by wearing devices and concerns regarding privacy protection. As a result, non-contact methods for monitoring vital signs have been proposed in recent years. In addition to avoiding the discomfort of long-term device wear, non-contact methods, particularly Frequency Modulated Continuous Wave (FMCW) radar, offer effective privacy protection compared to devices that utilize RGB cameras. The objective of this thesis is non-contact monitoring of vital signs. We propose a model primarily based on Temporal Convolutional Networks (TCN) to extract vital sign features. TCN enables Convolutional Neural Networks (CNN) to effectively model temporal sequences. By incorporating a subsystem with attention mechanisms, which provides relevant and informative cues, the model can monitor vital signs more effectively, thereby improving accuracy. |
顯示於類別: | [通訊工程研究所] 博碩士論文
|
文件中的檔案:
檔案 |
描述 |
大小 | 格式 | 瀏覽次數 |
index.html | | 0Kb | HTML | 30 | 檢視/開啟 |
|
在NCUIR中所有的資料項目都受到原著作權保護.
|
::: Copyright National Central University. | 國立中央大學圖書館版權所有 | 收藏本站 | 設為首頁 | 最佳瀏覽畫面: 1024*768 | 建站日期:8-24-2009 :::