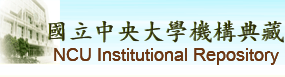 |
English
|
正體中文
|
简体中文
|
全文筆數/總筆數 : 80990/80990 (100%)
造訪人次 : 41636522
線上人數 : 1150
|
|
|
資料載入中.....
|
請使用永久網址來引用或連結此文件:
http://ir.lib.ncu.edu.tw/handle/987654321/93037
|
題名: | 中文訊息傳遞服務對話系統之建構;Construction of Message Deliver Service Dialog Systems: Schema Guided Dialogue Corpus Collection and Instruction-Guided Model Training |
作者: | 葉丞鴻;Yeh, Cheng-Hung |
貢獻者: | 資訊工程學系 |
關鍵詞: | 自然語言理解;機器學習;Schema-Guided Dialogue;語料建構;任務導向對話系統;Natural language understanding;Machine learning;Task-orient dialogue system;Dataset construction |
日期: | 2023-07-11 |
上傳時間: | 2024-09-19 16:39:08 (UTC+8) |
出版者: | 國立中央大學 |
摘要: | 對話系統,如客服系統、聊天機器人、智慧音箱等,是人工智能領域中受到廣泛期待的應用。任務型導向對話系統的挑戰包括訓練語料的收集、標記,以及模型的架構及訓練。在語料蒐集上,過去常使用 Wizard-of-Oz(WOZ) 方法,透過人與人之間的互動對話來進行語料的收集和標記。但由於使用 WOZ 方法時,標記人員須同時標記對話狀態及槽值,也必須留意先前對話歷史中是否提及某些實體,這會大大影響整體資料集的品質,且也無法迅速 建立對話語料。為了能加速資料的蒐集,部分研究人員也採用綱要引導對話 (Schema-Guided Dialogue,SGD) 來自動蒐集語料,透過定義清楚的綱要使對話代理來自動並蒐集對話。但由於綱要引導對話在自動對話蒐集前須做詳細的定義,且目前尚未有任何原始碼的公佈,使得研究人員現今仍普遍使用 WOZ來模擬人機互動的對話情境。 本文參照 Schema-Guided Dialogue(SGD) 的語料收集方法,透過使用者、助理兩個對話代理程式建立對話模擬器,建立一個能夠處理電子郵件、管理行事曆、以及傳遞訊息等三種服務的對話語料集。SGD 的做法為依據任務綱要(Schema) 來讓使用者代理能依據綱要提出需求,助理代理再查看綱要來向對方詢問相關資訊,或呼叫 API 來進行查詢。模擬器經過對話模擬後會產生由一連串對話行為組成的對話大綱,我們將對話大綱通過預先定義好的對話模板轉為樣版式句子,最後標記人員僅須結合多個單一句子轉成自然的對話,即可獲得訊息領域相關的對話語料 messageSGD。 基於本研究所建立的 messageSGD,我們以 T5 為基底套用至 TOD 對話 系統中的四個任務上,並在訓練時應用 Instruction Prompt 方法,在各個任務的輸入中添加任務敘述及特殊標記。經過如此的設計,使系統中各個模型能更理解四項任務的輸入輸出,在對話理解任務中整體準確度由 76.03 到 83.36,提升 7.33 個百分點,對話生成中的 BLEU-Score 也從 25.89 提升至 32.43。在對話狀態追蹤和對話決策任務上,我們也透過調整對話歷史的多寡,使狀態追蹤的準確度從 41.65 提升至 51.69,上升了 10.04 個百分點。;Dialogue systems, such as customer service systems, chatbots, and smart speakers, are widely anticipated applications in the field of artificial intelligence. The challenges of task-oriented dialogue systems include the collection and annotation of training data and the architecture and training of models. In terms of data collection, the Wizard-of-Oz (WOZ) method has often been used in the past, where conversational data is collected and annotated through interactive dialogues between humans. However, when using the WOZ method, annotators must pay attention to whether certain entities have been mentioned in the previous dialogue history. This significantly affects the overall quality of the dataset and hinders the rapid establishment of dialogue corpora. In this paper, we refer to the data collection method of Schema-Guided Dialogue (SGD), which involves building dialogue simulators using two dialogue agents. These simulators are designed to handle three types of services: email processing, calendar management, and message delivery. The SGD approach generates dialogue outlines based on task schema and then converts these outlines into templated sentences using pre-defined dialogue templates. As a result, annotators only need to combine multiple single sentences to create natural dialogues. Based on the messageSGD developed in this study, we apply the T5 model to four tasks in the TOD dialogue system. During training, we employ the Instruction Prompt method by adding task descriptions and special markers to the inputs of each task. Through this design, the models in the system gain a better understanding of the input and output for the four tasks, leading to improved performance. |
顯示於類別: | [資訊工程研究所] 博碩士論文
|
文件中的檔案:
檔案 |
描述 |
大小 | 格式 | 瀏覽次數 |
index.html | | 0Kb | HTML | 11 | 檢視/開啟 |
|
在NCUIR中所有的資料項目都受到原著作權保護.
|
::: Copyright National Central University. | 國立中央大學圖書館版權所有 | 收藏本站 | 設為首頁 | 最佳瀏覽畫面: 1024*768 | 建站日期:8-24-2009 :::