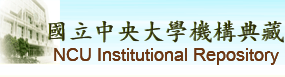 |
English
|
正體中文
|
简体中文
|
全文筆數/總筆數 : 80990/80990 (100%)
造訪人次 : 43999777
線上人數 : 1568
|
|
|
資料載入中.....
|
請使用永久網址來引用或連結此文件:
http://ir.lib.ncu.edu.tw/handle/987654321/94802
|
題名: | 研究多孔材料的孔徑分布:BJH方法的探討與機 器學習方法的應用潛力;Pore Size Distribution of Porous Materials: Evaluation of the BJH Method and Potential of Machine Learning Approaches |
作者: | 陳佑軒;Chen, You-Xuan |
貢獻者: | 化學工程與材料工程學系 |
關鍵詞: | 多孔材料;孔徑分析;BJH方法;吸附曲線;機器學習;神經網路;Porous material;Pore size distribution;BJH method;Adsorption isotherm;Machine learning;Neural network |
日期: | 2024-08-22 |
上傳時間: | 2024-10-09 15:30:53 (UTC+8) |
出版者: | 國立中央大學 |
摘要: | 多孔材料,如金屬有機框架(Metal-organicframework,MOF)和沸石(Zeolite),在氣體儲存、氣體分離和催化等各種應用中展現出顯著的潛力。而在眾多的物理性質中,孔徑分布(Poresizedistribution, PSD)是評估吸附相關應用能力的關鍵因素。到目前為止,有很多種方法已經被開發並用以測定孔徑分布,其中Barrett-Joyner-Halenda(BJH)方法是最為廣泛使用的一種,僅透過吸附或脫附等溫線便能快速地計算孔徑分布。儘 管有許多文獻指出使用BJH方法可能會低估狹窄介孔的孔徑分布,但目前為止,仍缺乏對BJH方法進行全面評估的研究。 因此,本研究探索了200多種具有不同孔洞結構的MOFs,使用蒙地卡羅(Grand canonical Monte Carlo, GCMC)方法模擬這些材料的吸附曲線,接著利用BJH方法計算這些結構的孔徑分布,以探討BJH方法決定孔徑分布的可行性。此外,本研究還建立了一系列以石墨烯為基礎的理想孔洞結構,包含圓柱形孔洞、狹縫型孔洞以及三角形孔洞,全方面探討孔洞的幾何形狀及表面化學如何影響吸附曲線的特徵及BJH的孔徑分布。由於已知這些材料的原子結構,因此可以使用Zeo++以幾何方法計算它們的孔徑分布作為比較基準。本研究通過詳細比較BJH方法和Zeo++計算的孔徑分布,系統 性地評估BJH方法的準確性。此部份的研究結果深入理解了使用BJH方法測定多孔材料孔徑分布的侷限性。在BJH方法的假設過於簡單的情況下,不同的孔洞幾何與原子組成都會嚴重影響BJH方法計算出來的孔徑分布,因此BJH方法便很難應用於擁有多樣化結構及原子組成的MOFs。 此外,本研究還利用機器學習(Machinelearning,ML)方法通過吸附曲線直接預測每個結構的孔徑分布。透過建立深度神經網路(Deepneuralnetwork,DNN)和卷積神經網路(Convolutional neural network, CNN)模型,藉由吸附曲線來預測孔徑分布,並將結果與BJH方法及Zeo++得到的孔徑分布進行比較。使用機器學習方法預測得到的孔徑分布比BJH方法計算得到的孔徑分布有更高的精確性,在多孔材料孔徑分布中取得了突破。 本研究不僅系統性評估了BJH方法的準確性,還展示了用機器學習方法在多孔材料孔徑分布預測中的潛力,為未來相關研究及多孔材料性質測定提供了新的思路。;Porous materials such as metal-organic frameworks (MOFs) and zeolites have shown sig nificant potential in various applications including gas storage, gas separation, and catalysis. Among their numerous properties, pore size distribution (PSD) plays a critical role in their per formanceinadsorption-related applications. Various methods have beendevelopedtodetermine PSD, with the Barrett-Joyner-Halenda (BJH) method [1] being widely recognized as one of the most renowned and extensively used techniques. The BJH method determines PSD from ad sorption or desorption isotherms. Despite reports indicating potential underestimation of PSD for narrow mesopores using the BJH method, a systematic assessment of its limitations and exploration of improvement measures remains relatively lacking. This study investigates over 200 structurally diverse MOFs to explore potential PSD under estimation by the BJH method. Additionally, a series of carbon-based structures with varying sizes were created as model systems to assess the geometric effects on PSD calculations. State of-the-art grand canonical Monte Carlo (GCMC) simulations were employed to compute argon adsorption isotherms of these structures, followed by BJH analysis to determine their PSD. Ge ometric methods implemented in the Zeo++ package [2] were used to calculate their PSD as benchmark references. Detailed comparisons between PSD obtained through BJH calculations and geometrically determined PSD provide a systematic evaluation of the accuracy of the BJH method. The results offer profound insights into understanding PSD determination in porous materials using the BJH method. Furthermore, efforts were made to develop a predictive model for PSD determination using machine learning (ML) approaches. Specifically, deep neural net work (DNN) and convolutional neural network (CNN) models were developed to predict PSDs from argon adsorption isotherms, achieving satisfactory performance. While challenges remain in achieving precise PSD predictions, the insights gained from this study contribute to method ological developments for PSD determination in porous materials. |
顯示於類別: | [化學工程與材料工程研究所] 博碩士論文
|
文件中的檔案:
檔案 |
描述 |
大小 | 格式 | 瀏覽次數 |
index.html | | 0Kb | HTML | 58 | 檢視/開啟 |
|
在NCUIR中所有的資料項目都受到原著作權保護.
|
::: Copyright National Central University. | 國立中央大學圖書館版權所有 | 收藏本站 | 設為首頁 | 最佳瀏覽畫面: 1024*768 | 建站日期:8-24-2009 :::