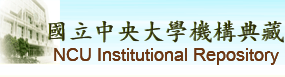 |
English
|
正體中文
|
简体中文
|
全文筆數/總筆數 : 80990/80990 (100%)
造訪人次 : 43370969
線上人數 : 1335
|
|
|
資料載入中.....
|
請使用永久網址來引用或連結此文件:
http://ir.lib.ncu.edu.tw/handle/987654321/95411
|
題名: | 基於深度學習之人形偵測以實現空中手寫與行人姿態辨識;Human Body Detection Based on Deep Learning to Facilitate Air Writing and Pedestrian Gait Recognition |
作者: | 賴慶榮;Lai, Chin-Rong |
貢獻者: | 資訊工程學系 |
關鍵詞: | 空中手寫;行人姿態;Air Writing;Pedestrian Gait |
日期: | 2024-06-27 |
上傳時間: | 2024-10-09 16:46:59 (UTC+8) |
出版者: | 國立中央大學 |
摘要: | 由於智慧型科技快速發展,人類姿態辨識的研究已成為熱門的研究領域之一。所謂姿態辨識即是使用電腦或智慧型設備來偵測並解譯人類姿態意涵的能力。這些姿態包括人類的手或軀體的移動、臉部表情甚或聲音指令等,皆可以做為用來控制設備或人機介面所使用。空中手寫是一種新型的人類與智慧型設備通信方法,允許使用者以自然連續的方式進行溝通控制。而步態辨識則是另一種健康照護或安全監視的應用領域,而最新興起的機器學習則可以應用於上述兩種技術的研究發展,並可對其所獲得的資料進行分析與解譯。 相較於其他書寫方法而言,空中手寫具有冗餘提筆筆畫、單一字書寫多樣性(multiplicity)及不同字軌跡類似模糊性(confusion)等獨特的特性,導致其較之於其書寫方法更具挑戰性。我們提出了一個嶄新的逆時序演算法,無需任何啟動的觸發動作或筆畫,有效率地過濾掉不必要的提筆筆畫,並簡化了複雜的筆劃軌跡比對程序。接著我們設計了一個三層階梯式結構,並以不同的取樣速率對空中手寫軌跡進行取樣,以解決書寫多樣性及軌跡類似模糊性等問題,所提出逆時序筆畫軌跡辨識的方法,其精確率可高達94%以上。 有關行人步態辨識方面,我們利用深度神經網路來達到自動偵測與辨識的功能。在抓取行人骨骼與關節移動部分,使用的是一連串的行人彩色影像輸入,而非使用穿戴式裝置來獲取影像資料。其後,我們使用捲機神經網路(CNN)抓取行人的位置,接著行人的密集光流這些低階特徵也被抽取出來,一起當成下階段處理的輸入資料。下一步是使用經微調的寬殘差網路(wide Residual Network)來抽取高階的抽象特徵。除此之外,為了克服使用二維(2D) CNN無法獲得局部且具有時序性特徵的困難,我們引入並使用了部分的三維(3D)卷積結構。此種設計使得在記憶體受到限制的實體環境中,能獲得有效的特徵抽取並提高了深度神經網路(DNN)的執行效能。實驗結果顯示本論文所提出的行人偵測辨識方法具有相當良好的執行效能。 ;With the rapid development of intelligent technologies, gesture recognition has become one of the most popular research areas in the world. It is the ability of a computer or smart device to detect and interpret human gestures. Such gestures, including movements of hand or body, facial expressions or even voice commands, can be used to control devices or interfaces. Air-writing is a new human and smart device communication approach which permits users to write inputs in a natural and relentless way. Gait recognition is another one for healthcare and surveillance. And machine learning can be applied to these two typical applications to analyze and interpret the captured data. Compared with other writing methods, air-writing is more challenging due to its unique characteristics such as redundant lifting strokes, multiplicity, and confusion. Without using any starting trigger, we propose a novel reverse time-ordered algorithm to efficiently filter out unnecessary lifting strokes, and thus simplifies the matching procedure. Then a tiered arrangement structure is proposed by sampling the air-writing results with various sampling rates to solve the multiplicity and confusion problems. The recognition accuracy of the proposed approach is satisfactorily higher than 94%. As to the gait recognition, we apply a deep neural network (DNN) to achieve gait-based automatic pedestrian detection and recognition. Instead of using wearable devices to precisely capture skeletal and joint movements, pedestrian color-image sequences are used as input. At a subsequent time, a pretraining convolutional neural network (CNN) is employed to capture pedestrian location, and the pedestrian dense optical flow is extracted to serve as concrete low-level feature inputs. Then, a finely-tuned DNN based on the wide residual network is employed to extract high-level abstract features. In addition, to overcome the difficulty of obtaining local temporal features by using a 2D CNN, part of the 3D convolutional structure is introduced into the CNN. This design enabled use of limited memory to acquire more effective features and enhance the DNN performance. The experimental results show that the proposed method has exceptional performance for pedestrian detection and recognition. |
顯示於類別: | [資訊工程研究所] 博碩士論文
|
文件中的檔案:
檔案 |
描述 |
大小 | 格式 | 瀏覽次數 |
index.html | | 0Kb | HTML | 26 | 檢視/開啟 |
|
在NCUIR中所有的資料項目都受到原著作權保護.
|
::: Copyright National Central University. | 國立中央大學圖書館版權所有 | 收藏本站 | 設為首頁 | 最佳瀏覽畫面: 1024*768 | 建站日期:8-24-2009 :::