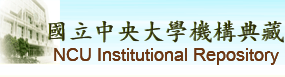 |
English
|
正體中文
|
简体中文
|
全文筆數/總筆數 : 80990/80990 (100%)
造訪人次 : 41631607
線上人數 : 4043
|
|
|
資料載入中.....
|
請使用永久網址來引用或連結此文件:
http://ir.lib.ncu.edu.tw/handle/987654321/95855
|
題名: | 基於人工智慧之晶圓瑕疵檢測採樣策略研究;Artificial Intelligent Based Sampling Strategy for Wafer Defect Inspection |
作者: | 林湧清;Lin, Yung-Cing |
貢獻者: | 機械工程學系 |
關鍵詞: | 人工智慧;聚類演算法;晶圓瑕疵採樣策略;Artificial Intelligent;Clustering Algorithms;Wafer Defect Sampling Strategy |
日期: | 2024-07-18 |
上傳時間: | 2024-10-09 17:20:27 (UTC+8) |
出版者: | 國立中央大學 |
摘要: | 在半導體製造過程中,確保晶圓的品質對於電子設備的性能和可靠性至 關重要。傳統的瑕疵採樣策略,如靜態和動態採樣,通常無法應對瑕疵檢測 中不斷變化的複雜性,導致檢測效率低下且人力成本高昂。為了解決這一問 題,本研究提出了一種利用機器學習技術的先進晶圓瑕疵檢測策略。該策略 採用了隨機森林(RF)和極限梯度提升(XGBoost)模型,結合合成少數過採樣 技術(SMOTE),並使用基於密度的空間聚類(DBSCAN)方法,將取樣對象 分為非群聚瑕疵和群聚瑕疵,從而提高瑕疵辨識的精確度和效率。對於非群 聚性瑕疵,使用改良式取樣增益圖來探討所需辨識瑕疵的機率閥值,對於群 聚性瑕疵,根據群聚內晶粒為瑕疵的機率做排序,取樣瑕疵機率最高的前五 點做人工複檢。 實驗證明,本研究所提出的採樣策略顯著降低了缺陷檢測點數,同時保 持了高水準的瑕疵召回率。對於非群聚瑕疵,當對瑕疵機率超過 20%的晶 粒進行取樣時,非群聚瑕疵的召回率達到 90%,顯示所提出的策略能有效 識別散佈於晶圓上的個別瑕疵。此外,對於群聚瑕疵,所提出的策略在幾乎 沒有瑕疵誤報率的情況下,以極少的取樣點數成功辨識了群聚內所有瑕疵。 對比於合作半導體廠商所開發的採樣演算法,本研究提出基於機器學習的 採樣策略在達到 90%瑕疵召回率的前提下,可以降低近 80%的總採樣點數。 這不僅顯著提升了檢測效率,還大幅減少了生產成本。因此,該採樣策略比 傳統方法更具效率,並且已具備在產線上實際應用的價值。 ;Ensuring the quality of wafers in semiconductor manufacturing is crucial for the performance and reliability of electronic devices. Traditional defect sampling strategies, such as static and dynamic sampling strategy, often fail to cope with the increasing complexity of defect detection, leading to inefficiencies and high labor costs. To address this issue, this study proposes an advanced wafer defect sampling strategy leveraging machine learning techniques. The proposed strategy employs Random Forest (RF) and Extreme Gradient Boosting (XGBoost) models, combined with the Synthetic Minority Over-sampling Technique (SMOTE), and utilizes the Density-Based Spatial Clustering of Applications with Noise (DBSCAN) method to classify defects into non-clustered and clustered categories, thereby enhancing the accuracy and efficiency of defect identification. For non-clustered defects, the Modified Sampling Gains Chart is used to determine the probability threshold required for defect identification. For clustered defects, the defects are ranked based on the probability of the dies within the cluster being defective, and the top five dies with the highest defect probability are sampled. Validation experiments demonstrate that the proposed strategy significantly reduces the number of sampling dies while maintaining a high recall rate of defect. For non-clustered defects, when sampling dies with a defect probability exceeding 20%, the recall rate for non clustered defects reaches 90%. This indicates that the proposed strategy effectively identifies individual defects across the wafer. Additionally, for clustered defects, the proposed strategy successfully identifies all defects within the clusters with minimal false positives, using a low number of sampling points. Compared to the sampling algorithms developed by the collaborative semiconductor manufacturer, the machine learning-based sampling strategy proposed in this study achieves a 90% defect recall rate while reducing the total number of sampling points by nearly 80%. This not only significantly enhances detection efficiency but also substantially reduces production costs. Consequently, this sampling strategy is more efficient than traditional methods and demonstrates practical applicability in production lines. |
顯示於類別: | [機械工程研究所] 博碩士論文
|
文件中的檔案:
檔案 |
描述 |
大小 | 格式 | 瀏覽次數 |
index.html | | 0Kb | HTML | 28 | 檢視/開啟 |
|
在NCUIR中所有的資料項目都受到原著作權保護.
|
::: Copyright National Central University. | 國立中央大學圖書館版權所有 | 收藏本站 | 設為首頁 | 最佳瀏覽畫面: 1024*768 | 建站日期:8-24-2009 :::