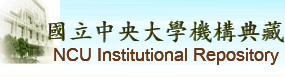 |
English
|
正體中文
|
简体中文
|
全文筆數/總筆數 : 80990/80990 (100%)
造訪人次 : 41143720
線上人數 : 210
|
|
|
資料載入中.....
|
請使用永久網址來引用或連結此文件:
http://ir.lib.ncu.edu.tw/handle/987654321/86220
|
題名: | 類神經網路逆向工程理解遙測資訊:以Landsat 8植被分類為例;A Case Study Using Landsat 8 Images for Vegetation Classification |
作者: | 史楷德;Sikhondze, Nhlakanipho |
貢獻者: | 國際永續發展碩士在職專班 |
關鍵詞: | 分類;人工神經網絡;歸一化植被指數;Classification;Artificial neural network;Landsat 8;normalized difference vegetation index (NDVI) |
日期: | 2021-10-12 |
上傳時間: | 2021-12-07 12:18:08 (UTC+8) |
出版者: | 國立中央大學 |
摘要: | 人工神經網路 (ANN) 廣泛用於多種用途,其中之一是衛星影像的地表覆蓋分類。然而,人們對人工神經網路如何在輸入和輸出之間建立聯繫知之甚少。本研究的目的是獲得基於 ANN權重的數學方程式,從中推斷輸入和輸出之間的關係,進而剖析ANN了解遙測資訊的方式。 在本研究中獲得並使用了不同植被覆蓋量的三個資料集(Landsat 8 影像)。分別使用ENVI 5.3、ArcMap 10.5和Matlab對這三個資料集進行了預處理。神經網路是在Python 3.7上構建和研究的。每個神經網路都以DeepLIFT演算法分析其認為最重要的輸入並重複訓練。迭代過程中,最不重要的頻段將被刪除,從而使最終輸入數量少於最初的11個頻段。下一步驟對ANN進行修剪,刪除被認為是多餘的節點/神經元,同時保持ANN精度相對不變,進而可提升處理效能及便於進行數學方程萃取。最終,根據修剪後的ANN權重和偏差進行統整合併以萃取其極簡化之數學方程。此數學方程則可在Landsat 8影像中識別植被,如同正規化差異植生指標(NDVI)之角色。 預剪枝人工神經網絡(pre-pruned ANNs)的準確率分別為 96%、95% 和 92%,在剪枝後,經過逆向工程程序,它們的準確率保持不變。經過逆向工程,它們相應的 ANN 派生方程執行時間與各自研究領域相關的 NDVI 確定的執行時間相當。圖像中的土地覆蓋類型會影響 ANN 如何在輸入和輸出之間建立關係。這些不同的關係最終導致不同的波段成為逆向工程方程中的變量。就植被分類而言,人工神經網絡受數據本身性質的影響很大。基於逆向工程方程,Coastal 氣溶膠、熱紅外和短波紅外波段在確定非植被(即裸地、已建成的土地和水體)方面發揮了作用,而近紅外波段與植被像素的確定呈正相關。 ;Artificial neural networks (ANNs) are used for many purposes, one of which is the land cover classification of satellite images. However, little is known about how the ANNs make connections between inputs and outputs. The aim of this study was to acquire mathematical equations based on the weights of an ANN, from which a relationship between inputs and outputs could be inferred. Three identical ANNs were constructed (20 hidden layers, ReLU activation functions for neurons in the hidden layers and a sigmoid function in the output layer) for three distinct study areas, using vegetation-detection as a test case. The first major step was training each neural network while eliminating the inputs that they deemed to be the least important, via the DeepLIFT algorithm. The least important bands were removed thus leaving a certain number of inputs which was less than the original 11 bands in each case. The second step involved condensing the ANNs by removing redundant neurons leading to the improvement of execution times while keeping the ANNs’ accuracies relatively unchanged. The third major step was the formulation of three separate equations by reverse-engineering the ANNs. The three datasets (Landsat 8 OLI TIRS images) had different types of land coverage. Independently, these three datasets were preprocessed using ENVI 5.3, ArcMap 10.5 and Matlab. The neural networks were constructed and studied on Python 3.7. The pre-pruned ANNs had accuracies of 96%, 95% and 92% and, upon being pruned, their accuracies remained relatively unchanged after reverse engineering procedure. Having been reverse-engineered, their corresponding ANN-derived equations had comparable execution times to that of the NDVI determination relevant to their respective study areas. The land cover type in an image has an effect on how an ANN makes relationships between inputs and outputs. These different relationships ultimately result in different bands being variables in the reverse-engineered equations. As far as vegetation classification is concerned, ANNs are heavily influenced by the nature of the data itself. Based on the reverse-engineered equations, Coastal Aerosol, Thermal Infrared and Short Wave Infrared bands play a role in the determination of non-vegetation, i.e. bare land, built up land and water bodies while the Near Infrared band has a positive correlation to the determination of pixels with vegetation. |
顯示於類別: | [國際永續發展在職專班] 博碩士論文
|
文件中的檔案:
檔案 |
描述 |
大小 | 格式 | 瀏覽次數 |
index.html | | 0Kb | HTML | 130 | 檢視/開啟 |
|
在NCUIR中所有的資料項目都受到原著作權保護.
|
::: Copyright National Central University. | 國立中央大學圖書館版權所有 | 收藏本站 | 設為首頁 | 最佳瀏覽畫面: 1024*768 | 建站日期:8-24-2009 :::