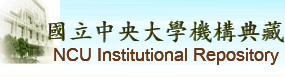 |
English
|
正體中文
|
简体中文
|
全文筆數/總筆數 : 80990/80990 (100%)
造訪人次 : 41266341
線上人數 : 214
|
|
|
資料載入中.....
|
請使用永久網址來引用或連結此文件:
http://ir.lib.ncu.edu.tw/handle/987654321/93842
|
題名: | 人工智慧應用於石英晶圓放電加工品質之預測;Application of Artificial Intelligence for Predicting Machining Quality in Electrical Discharge Machining of Quartz Wafer |
作者: | 郭咨妤;Kuo, Tzu-Yu |
貢獻者: | 機械工程學系 |
關鍵詞: | 放電加工;人工智慧;石英晶圓;Electrical Discharge Machining (EDM);Artificial Intelligence(AI);quartz wafer |
日期: | 2023-07-31 |
上傳時間: | 2024-09-19 17:41:28 (UTC+8) |
出版者: | 國立中央大學 |
摘要: | 放電加工(EDM)是一種極為成熟的技術,其非接觸式加工方式具有明顯的優勢,已被廣泛應用於金屬加工的各個領域中。近期,在石英振盪器封裝上使用了新型的晶圓級封裝技術,並且將封裝的材料從原本的陶瓷蓋板改為石英晶圓。然而,由於石英晶圓的非導電特性,無法直接進行EDM加工。因此,本研究採用輔助電極的方法,賦予石英晶圓表面導電性,以實現對硬脆石英晶圓的EDM加工。同時,本研究開發了有效且可靠的深度神經網路(DNN)模型,對EDM加工後的加工品質進行預測和優化。 在本研究中,首先選擇了四個可調整的加工參數進行了81組的全因子實驗。接著,利用隨機森林回歸演算法(RFRM)以及響應表面演算法(RSM)確認了這四個加工參數對加工品質的顯著影響,並適用於作為DNN預測模型的輸入參數。本研究透過粒子群演算法(PSO)來決定DNN預測模型的架構,並藉由五摺交叉驗證方法進行微調,獲得了一個具有四層隱藏層的最佳DNN預測模型。所建立的DNN模型在加工品質(包括鑽孔入口及出口直徑)的預測展現出良好表現,無論在數據訓練集、驗證集及測試集的預測上,都具有極低的絕對百分比誤差值(MAPE)和非常高的決定係數值(R2)。進一步將此DNN預測模型結合PSO,找出可產生出最佳加工品質的最佳化加工參數組合並進行實驗驗證。實驗結果與模型預測的最佳加工品質有良好的一致性,證明了本研究所建立以人工智慧為基礎的模型具有高效性及可靠性,能夠在石英晶圓上實現最佳的放電加工鑽孔品質。 ;Electrical Discharge Machining (EDM) is a highly mature technology widely used in various domains of metal processing. Its non-contact machining approach offers significant advantages. Recently, a novel wafer-level packaging technique has been employed in the packaging of quartz oscillator wafers. However, direct EDM drilling was not feasible for the quartz oscillator wafer due to its non-conductive characteristics. Hence, this study used the method of assisting electrode to impart conductivity to the surface of quartz wafer, enabling EDM drilling of the hard and brittle quartz wafer. Additionally, an effective Deep Neural Network (DNN) model was developed to predict and optimize the EDM machining quality. In this study, a total of eighty-one sets of full-factorial experiments were conducted by selecting four adjustable machining parameters, namely Ton, Toff, LV, and GAP. The significant effects of these four machining parameters on the machining quality were analyzed and confirmed using the Random Forest Regression Model (RFRM) and the Response Surface Method (RSM). They were then appropriately used as the input parameters of the DNN predictive model. The Particle Swarm Optimization (PSO) algorithm was employed to determine the architecture of the DNN predictive model, which was further fine-tuned through five-fold cross-validation (CV), resulting in an optimal DNN model with four hidden layers. The established DNN model exhibited excellent performance in predicting the machining quality, including the inlet and outlet diameters, with extremely low Mean Absolute Percentage Error (MAPE) and very high Coefficient of Determination (R2) for the training, validation, and testing datasets. Subsequently, this predictive model was combined with PSO to find the optimal combination of machining parameters for achieving the best machining quality. The good agreement between the validation experiments and the predictions of optimal machining quality demonstrates the effectiveness and reliability of the developed artificial-intelligent based model in achieving optimal EDM drilling quality of quartz wafers. |
顯示於類別: | [機械工程研究所] 博碩士論文
|
文件中的檔案:
檔案 |
描述 |
大小 | 格式 | 瀏覽次數 |
index.html | | 0Kb | HTML | 12 | 檢視/開啟 |
|
在NCUIR中所有的資料項目都受到原著作權保護.
|
::: Copyright National Central University. | 國立中央大學圖書館版權所有 | 收藏本站 | 設為首頁 | 最佳瀏覽畫面: 1024*768 | 建站日期:8-24-2009 :::