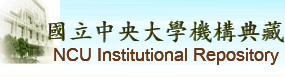 |
English
|
正體中文
|
简体中文
|
全文筆數/總筆數 : 80990/80990 (100%)
造訪人次 : 41142282
線上人數 : 335
|
|
|
資料載入中.....
|
請使用永久網址來引用或連結此文件:
http://ir.lib.ncu.edu.tw/handle/987654321/95665
|
題名: | 利用深度學習基於時間與空間特徵生成人體姿態骨架;Use deep learning to generate human posture skeleton based on temporal and spatial features |
作者: | 謝瑞筑;Hsieh, Jui-Chu |
貢獻者: | 資訊工程學系 |
關鍵詞: | 無線訊號;人體姿態估計;深度學習 |
日期: | 2024-07-29 |
上傳時間: | 2024-10-09 17:08:01 (UTC+8) |
出版者: | 國立中央大學 |
摘要: | 在最近這幾年來,人體姿態估計的發展越發成熟,在醫療場景、手勢辨識、頭部運 動軌跡等運用,除了大家最常見的電腦視覺領域,使用無線訊號作為人體姿態估計 也開始有越來越多的應用,不過使用電腦視覺有一個很重大的問題:隱私。假設當 我們今天需要在一個養老院或是醫院裡的病房監督老人或是病患的狀況時,使用 傳統方式的攝影機去紀錄房間內人員會使得他們失去隱私,也擔心這些影像可能 被不肖人士拿去做違法的不良行為,因此我們考慮到了WiFi在現今生活的使用率 很高且幾乎遍地都是,利用WIFI的無線電波的特性,我們使用了WIFI的實體層訊 號CSI(Channel state information)來獲得空間中活動的人體骨架姿態。WiFi的CSI訊 號會反射空間中的任何物體,導致訊號會參雜很多空間中的雜訊,這些雜訊會使得 我們的深度學習網路所生成的骨架精準度不夠高,尤其是在四肢的效果是最差的, 所以我們的資料集對於CSI的振幅以及相位做了去噪的處理。 為了可以生成出精確的人體姿態,我們考慮到CSI訊號是一個時間序列,並且人 體動作間的前後關係對波形也有影響,所以我們使用了時間序列網路TCN來獲取時 間特徵,再利用Attention UNET從時間特徵與空間特徵生成人體骨架關節點座標。 我們的成果在PCK@50達到了97%的準確度。;In recent years, human posture estimation has advanced significantly, with applications in medical scenarios, gesture recognition, and head motion tracking. While computer vision is commonly used, it raises privacy concerns, especially in settings like nursing homes or hospital rooms, where camera monitoring can invade privacy and risk misuse of images.Given the widespread use of WiFi, we utilize its physical layer signal, Channel State Information (CSI), to capture human skeleton postures. WiFi’s CSI signals reflect off various objects, including human bodies, causing noise that affects the accuracy of the generated skeletons, particularly the limbs. To address this, we applied noise reduction techniques to the amplitude and phase of the CSI signals in our dataset. To generate accurate human posture, we consider that CSI signals are time series data, and the sequential relationship between human movements affects the waveform. Therefore, we used a Temporal Convolutional Network (TCN) to capture temporal features and then utilized an Attention UNET to generate human skeleton joint coordinates from both temporal and spatial features. Our results achieved an accuracy of 97% in PCK@50. |
顯示於類別: | [資訊工程研究所] 博碩士論文
|
文件中的檔案:
檔案 |
描述 |
大小 | 格式 | 瀏覽次數 |
index.html | | 0Kb | HTML | 33 | 檢視/開啟 |
|
在NCUIR中所有的資料項目都受到原著作權保護.
|
::: Copyright National Central University. | 國立中央大學圖書館版權所有 | 收藏本站 | 設為首頁 | 最佳瀏覽畫面: 1024*768 | 建站日期:8-24-2009 :::