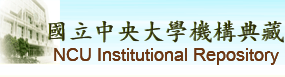 |
English
|
正體中文
|
简体中文
|
全文筆數/總筆數 : 81570/81570 (100%)
造訪人次 : 47065289
線上人數 : 453
|
|
|
資料載入中.....
|
請使用永久網址來引用或連結此文件:
http://ir.lib.ncu.edu.tw/handle/987654321/84697
|
題名: | 基於Transformer架構之開放式對話系統探討;Transformer-Based Models for Open Dialogue Systems |
作者: | 柯士文 |
貢獻者: | 資訊管理學系 |
關鍵詞: | 開放式對話系統;文字生成;注意力機制;語言模型;自動評量方式;對話系統應用;Open dialogue systems;text generation;attention mechanism;language models;evaluation methods;applications of dialogue systems |
日期: | 2020-12-08 |
上傳時間: | 2020-12-09 10:44:36 (UTC+8) |
出版者: | 科技部 |
摘要: | 近年許多研究提出各種基於神經網路的對話系統,但模擬對話仍然是對話生成領域中最棘手的挑戰之一。對話系統以對話能力可分為主要兩種形式。第一個是任務導向或close-domain的對話系統,它的對話能力有限,但針對執行特定於任務時會非常有用任務導向的模型是為了完成特定的任務,例如智慧客服等。第二種對話系統是非任務導向或open-domain開方式對話系統。此對話系統試圖模仿人類真實的對話,但目前的模型仍然無法達到對話的順暢,因為使用者不一定有明確的意圖。而大多對話系統與其相關研究仍採用基於RNN架構Seq2Seq模型,此外Transformer在Neural Machine Translation (NMT) 領域上的表現遠超於基於RNN架構的Seq2Seq模型,但鮮少研究將基於RNN的Seq2Seq模型和Transformer模型在對話生成領域上進行評估和比較。此外,機器翻譯與對話系統常用的自動評估指標,如BLEU、Perplexity等,都無法有效套用在開放式對話系統的評量。如(Liu et al., 2016b) 提出評估指標在使用閒聊性質的資料集訓練模型時和人工判斷是呈現弱相關。因此本計畫會採用基於RNN的Seq2Seq模型和Transformer為基底的模型,並使用對話相關資料集進行對模型進行訓練。本計畫將著重在於開放式對話這個任務進行探討,並且使用多種量化分析指標和質性分析來證實二者模型架構對於open-domain對話生成領域中的合適性。人工評估在對話系統中是一項重要的評估方法,但如何執行人工評估在各研究中存在著許多差異,也沒有固定標準。因此本計畫希望透過分析各個自動評估方式和人工評估之間的相依性和可靠性,進而推薦有效自動評估方式的通用公式,期待對開放式對話系統之評量方法做出貢獻。最後,本計畫部分成果預計延伸至本人目前正在執行的科技部產學計畫,進一步開發精準行銷與智慧行銷客服等應用。 ;In recent years, many studies have proposed various neural network-based dialog systems, but generating convincing dialogs is still one of the most challenging tasks in the field of dialog generation. Dialogue systems can be divided into two categories. The first is a task-oriented or close-domain dialogue system, which has limited dialogue capabilities, but is task-oriented for performing specific tasks, such as a smart kiosk or customer service. The second type of dialogue system is a non-task-oriented or open-domain dialogue system. An open dialogue system aims to imitate the real human conversation, however the current techniques still cannot deliver human-like dialogue smoothly due to the fact that the user does not necessarily have a clear intention when engaging in an open conversation. Most dialogue systems and related work investigated the Sequence-to-sequence model based on the RNN architecture. Recent studies have shown that the Transformer model outperformed the Seq2Seq models in Neural Machine Translation (NMT) tasks, but the investigation on Transformer-based models for open dialogue systems is still lacking.Furthermore, the automatic evaluation metrics commonly used in machine translation and dialogue systems, such as BLEU and Perplexity, are inadequate for the evaluation of open dialogue systems. For example, Liu et al. (2016) showed that the evaluation metrics show a weak correlation with human judgment when training models using conversational data sets. Therefore, this project will use the RNN-based Seq2Seq model and the Transformer-based model, and use dialog-related data sets to train the model. This project will focus on the task of open dialogue, and use a variety of quantitative evaluation metrics as well as qualitative analysis to verify the suitability of the two model architectures in the field of open-domain dialogue generation. Human evaluation is an important evaluation method in a dialogue system, but many differences were observed in how to perform manual evaluation in various studies. We plan to analyze the interdependence and reliability between each automatic evaluation metrics and human evaluation. Finally, we plan to apply the research outcomes of this project to our ongoing 科技部產學計畫 where applications such as precision marketing and intelligent customer service are in discussion. |
關聯: | 財團法人國家實驗研究院科技政策研究與資訊中心 |
顯示於類別: | [資訊管理學系] 研究計畫
|
文件中的檔案:
檔案 |
描述 |
大小 | 格式 | 瀏覽次數 |
index.html | | 0Kb | HTML | 219 | 檢視/開啟 |
|
在NCUIR中所有的資料項目都受到原著作權保護.
|
::: Copyright National Central University. | 國立中央大學圖書館版權所有 | 收藏本站 | 設為首頁 | 最佳瀏覽畫面: 1024*768 | 建站日期:8-24-2009 :::