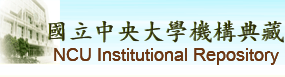 |
English
|
正體中文
|
简体中文
|
Items with full text/Total items : 78852/78852 (100%)
Visitors : 35494506
Online Users : 234
|
|
|
Loading...
|
Please use this identifier to cite or link to this item:
http://ir.lib.ncu.edu.tw/handle/987654321/86711
|
Title: | 應用對抗式Reptile於家電產品網路評論之研究;Home Appliance Review Research Via Adversarial Reptile |
Authors: | 甘岱融;Kan, Tai-Jung |
Contributors: | 資訊工程學系 |
Keywords: | 元學習;遷移式學習;意見目標情緒分析;meta learning;transfer learning;ABSA |
Date: | 2021-08-18 |
Issue Date: | 2021-12-07 13:08:49 (UTC+8) |
Publisher: | 國立中央大學 |
Abstract: | 對於生產家電產品的廠商來說,自家所推出的產品在社群平台上討論及喜好程度是必須要蒐集的資訊。透過網路評價所給出的回饋,能即時反應該廠牌的產品是否為大眾所接受,更可以進一步分析出產品的何項資訊是較為人所討論,在後續製造新產品的過程中,可以揚長補短,改善自家產品。
本論文方法總共分為兩部分,在第一部分時,我們將人工標記好的家電資料分成三個子任務:命名實體辨識(Name Entity Recognition)、目標種類探索(Aspect Category Extraction)、情緒分類(Sentiment Classification)。在NER的任務中使用BERT-BiLSTM-CRF的模型,而ACE、SC則參考Sun等人的做法,將輸入加入輔助句子的資訊,並使用BERT為基礎的分類模型,用上述方法在三個任務中得出了這些任務的基本效能。在第二部分,在SC的任務中,嘗試針對不同的目標種類訓練任務導向的模型,目標要提升SC的基礎效能。此部分我們結合遷移式學習中,元學習的Reptile演算法及對抗式訓練(Adversarial Training)的概念組成的對抗式Reptile算法。希望能藉由元學習中少樣本學習的優勢以及對抗式訓練的架構組合出一個可以在各種分布的資料中都可以快速訓練好的模型。
本研究擷取社群網站上家電相關產品的討論文章並進行人工標記,再從中對半切出訓練及測試資料集。就研究結果顯示,SC的任務中,在未加入任何遷移式學習的方法下,對於不同目標種類訓練各自的模型的Macro-F1低於基準值(60.1\% v.s. 68.6\%)。而使用了對抗式Reptile架構訓練後的模型,其Macro-F1進步至70.3\%。顯示遷移式學習有助於SC的任務提升其效能。;For manufacturers of home appliances, the overview and preference of their products on social media is the information that must be collected. The feedback given through the online evaluation can instantly reflect whether the products are acceptable to others, and it can further analyze what information about the product is more discussed. It can make up for the shortcomings and improve their own products in the future.
The method in this paper is divided into two parts. In the first part, we divide the manually labeled home appliance data into 3 subtasks: Named Entity Recognition, Aspect Category Extraction, and Sentiment Classification. In the NER task, we use BERT-BiLSTM-CRF model.In ACE, SC tasks, we refer to Sun et al., adding the information of the auxiliary sentence, and using the BERT classification model. We use the above method to get the basic performance of these 3 tasks. In the second part, in the SC task, we try to train task-oriented models for different aspect category. The goal is to improve the basic performance of SC. In this part, we combine the Reptile algorithm of meta learning and adversarial training concepts to propose an adversarial Reptile algorithm. We hope to combine the advantages of few-shot learning in meta learning and the structure of adversarial training framework to create a model that can be quickly trained on various distributed data.
This research extracts reviews on social media about home appliance and manually labeled them, then cut out training and testing data sets in half. The result shows that in the SC task, without any transfer learning method, the Macro-F1 of training task-oriented models for different aspect category is lower than the benchmark(60.1\% v.s. 68.6\%).After using the adversarial Reptile architecture training model, the Macro-F1 has improved to 70.3\% in task-oriented models. It shows that transfer learning method is helpful for SC task. |
Appears in Collections: | [Graduate Institute of Computer Science and Information Engineering] Electronic Thesis & Dissertation
|
Files in This Item:
File |
Description |
Size | Format | |
index.html | | 0Kb | HTML | 129 | View/Open |
|
All items in NCUIR are protected by copyright, with all rights reserved.
|
::: Copyright National Central University. | 國立中央大學圖書館版權所有 | 收藏本站 | 設為首頁 | 最佳瀏覽畫面: 1024*768 | 建站日期:8-24-2009 :::