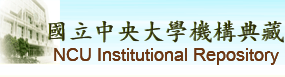 |
English
|
正體中文
|
简体中文
|
全文筆數/總筆數 : 80990/80990 (100%)
造訪人次 : 41623851
線上人數 : 831
|
|
|
資料載入中.....
|
請使用永久網址來引用或連結此文件:
http://ir.lib.ncu.edu.tw/handle/987654321/95564
|
題名: | Rephrasing Human Instructions for Instruction-tuned LLMs |
作者: | 盧俊吉;Lu, Jyun-Ji |
貢獻者: | 資訊管理學系 |
關鍵詞: | 指令跟隨;離散提示;改寫;黑盒優化;instruction following;discrete prompt;paraphrasing;black-box optimizing |
日期: | 2024-07-26 |
上傳時間: | 2024-10-09 17:03:40 (UTC+8) |
出版者: | 國立中央大學 |
摘要: | 生成式AI服務 ( ChatGPT、Gemini 和 Copilot ),因其能夠遵循人類指令並生成相對應的回應而受到廣泛的關注。這些大型語言模型 (LLMs) 的人類指令遵循能力主要是來自於指令調整 ( Instruction Tuning ),該方法透過指令跟隨資料集以監督式微調 (SFT) 的方式訓練LLMs。然而,研究顯示,經過指令調整的LLMs ( Instruction-tuned LLMs )對離散文本的擾動仍然具有一定敏感性,可能導致不可預測、無法控制的生成行為,進而影響遵循人類指令的表現。鑒於通用生成式AI服務的大量推出,是否可以改善人類直覺的指令輸入,以符合Instruction-tuned LLMs的偏好,實現穩定、可控且高品質的回應,同時解決用戶對如何撰寫精確指令的困擾。 優化離散文本以迎合LLMs偏好的概念,已在離散提示工程 (Discrete prompt engineering) 的研究中證實其在傳統NLP任務中的有效性。然而,與傳統NLP任務的資料不同,人類指令源自於人類現實世界中的互動,高度使用者友好且複雜,直接應用先前離散提示工程的方法於人類指令並不實際。 在我們的實驗當中,我們展示了我們提出的方法可以通過自動改寫人類指令以增強instruction-tuned LLMs生成回應的表現。這樣表現的提升在多樣性越高的訓練資料上更加的明顯。此外我們也觀察到相同的指令改寫方法可以泛化到具有相同主幹的instruction-tuned LLMs,而具有不同主幹的instruction-tuned LLMs對於離散文本的偏好可能不同。我們的方法展示了在離散層級和黑箱情境下改善instruction-tuned LLMs表現的可行性,同時保持人類指令的語義一致性和可解釋性。 ;Generative AI services like ChatGPT, Gemini, and Copilot have gained significant attention for their ability to follow human instructions and assist with real-world tasks. The core mechanism behind their effectiveness is instruction tuning — a process involving supervised fine-tuning (SFT) with paired datasets of human instructions and responses. Despite the ability of following human instructions from instruction-tuned large language models (LLMs), studies still show that instruction-tuned LLMs exhibit sensitivity to perturbations in discrete text, which can cause the unpredictable, uncontrollable generation behavior and may lead to performance degradation. Given the emergence of general-purpose generative AI services, whether can human instructions be optimized to align with the preferences of instruction-tuned LLMs for stable, controllable and high-quality responses generation while also addressing users′ concerns about crafting precise instructions. The concept of enhancing LLMs’ performance by optimizing discrete text to cater LLMs’ preference has already shown the effectiveness at discrete prompt engineering, which enhancing the performance of LLMs on traditional NLP tasks by finding optimal discrete templates or texts. However, unlike traditional NLP tasks, human instructions are user-friendly, highly variable, and derived from real-world interactions, making direct application of previous discrete prompt methods to human instructions impractical. In our experiments, we demonstrate that our proposed method enhances the response quality of instruction-tuned LLMs simply by rephrasing human instructions. This enhancement is more pronounced with a richer variety of training data. Additionally, we observe that the same optimization approach applies across instruction-tuned LLMs sharing the same backbone, whereas instruction-tuned LLMs with different backbones may have different preferences for discrete text. Our method showcases the feasibility of improving instruction-tuned LLMs at the discrete level and in a black-box scenario, while maintaining the semantic consistency and explainability of human instructions. |
顯示於類別: | [資訊管理研究所] 博碩士論文
|
文件中的檔案:
檔案 |
描述 |
大小 | 格式 | 瀏覽次數 |
index.html | | 0Kb | HTML | 38 | 檢視/開啟 |
|
在NCUIR中所有的資料項目都受到原著作權保護.
|
::: Copyright National Central University. | 國立中央大學圖書館版權所有 | 收藏本站 | 設為首頁 | 最佳瀏覽畫面: 1024*768 | 建站日期:8-24-2009 :::