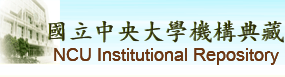 |
English
|
正體中文
|
简体中文
|
全文筆數/總筆數 : 81570/81570 (100%)
造訪人次 : 48531064
線上人數 : 1520
|
|
|
資料載入中.....
|
請使用永久網址來引用或連結此文件:
http://ir.lib.ncu.edu.tw/handle/987654321/95669
|
題名: | 基於質譜儀資料使用深度學習方法預測不同地區之耐甲氧西林金黃色葡萄球菌之抗藥性 |
作者: | 高士唐;Kao, Shi-Tang |
貢獻者: | 資訊工程學系 |
關鍵詞: | 金黃色葡萄球菌;基質輔助雷射脫附電離飛行時間質譜;深度學習;抗生素抗藥性;Staphylococcus aureus;MALDI-TOF;Deep Learning;Antibiotic Resistance |
日期: | 2024-07-29 |
上傳時間: | 2024-10-09 17:08:09 (UTC+8) |
出版者: | 國立中央大學 |
摘要: | 耐甲氧西林金黃色葡萄球菌(Methicillin-Resistant Staphylococcus aureus,MRSA)於1961年在英國首次被識別,是一種對多種抗生素具有抗藥性的細菌,並且有著極高的死亡率和發病率,因此也被稱為“超級細菌”。基質輔助雷射脫附電離飛行時間質譜法(MALDI-TOF MS)提供了一種更快速的微生物鑑定替代方法,當與機器學習結合使用時,能顯著提高微生物鑑定的準確性和效率。不同地理區域的MRSA菌株展示出特定的亞型分布,先前研究多集中於單一地區的MRSA抗藥性預測,而忽略了地理差異對於抗藥性模式的影響,導致模型性能會因為MRSA資料集來源的差異性而有所下降。為了解決這一問題,在本研究中我們使用超過25,000個來自台灣和瑞士的數據樣本,採用深度學習方法並結合聯邦式學習和遷移式學習,對模型進行了跨地區訓練以提升模型的適應性和準確性。結果顯示在獨立測試集的AUROC和AUPRC分別達到了0.82以及0.7以上。我們還通過shapley值和差異表達分析鑑定了幾個與MRSA特有峰值相關的關鍵特徵,比如2410至2419範圍內的2415點代表PSM-mec、6590至6599範圍內的6593點代表SA1452蛋白並進行了台灣和瑞士抗藥性與敏感性樣本之間的比較。最後以宏觀的角度探討了全球MRSA亞型的分布和盛行率,發現與我們的結果相關聯,如台灣的ST59-V和ST239-III,以及瑞士或歐洲的ST22-IV。這些成果不僅豐富了我們對MRSA全球分布的了解,也強調了利用地區化數據提升抗藥性預測準確性的重要性。;Methicillin-Resistant Staphylococcus aureus (MRSA) was first identified in the UK in 1961. It is a type of bacteria resistant to multiple antibiotics and has a very high mortality and morbidity rate, earning it the label of a “superbug.” Matrix-Assisted Laser Desorption Ionization Time-of-Flight Mass Spectrometry (MALDI-TOF MS) provides a faster alternative for microbial identification. When combined with machine learning, it significantly enhances the accuracy and efficiency of microbial identification. MRSA strains from different geographic regions exhibit specific sequence types distribution. Previous studies have primarily focused on predicting MRSA resistance within a single region, neglecting the impact of geographic differences on resistance patterns, leading to decreased model performance due to differences in the sources of MRSA datasets. To address this issue, our study utilized over 25,000 data samples from Taiwan and Switzerland. We employed deep learning methods combined with federated learning and transfer learning to train the model across regions, improving its adaptability and accuracy. The results showed that the AUROC and AUPRC in the independent test sets reached over 0.82 and 0.7, respectively. Through shapley value and differential expression analysis, we identified several key features associated with MRSA specific peaks, such as the 2415 peak in the 2410-2419 range representing PSM-mec and the 6593 peak in the 6590-6599 range representing the SA1452 protein, and conducted a comparative analysis of resistance and susceptible samples from Taiwan and Switzerland. Finally, from a macroscopic perspective, we explored the global distribution and prevalence of MRSA sequence types, finding correlations with our results, such as ST59-V and ST239-III in Taiwan and ST22-IV in Switzerland or Europe. These findings not only enrich our understanding of the global distribution of MRSA but also emphasize the importance of using localized data to improve the accuracy of resistance predictions. |
顯示於類別: | [資訊工程研究所] 博碩士論文
|
文件中的檔案:
檔案 |
描述 |
大小 | 格式 | 瀏覽次數 |
index.html | | 0Kb | HTML | 80 | 檢視/開啟 |
|
在NCUIR中所有的資料項目都受到原著作權保護.
|
::: Copyright National Central University. | 國立中央大學圖書館版權所有 | 收藏本站 | 設為首頁 | 最佳瀏覽畫面: 1024*768 | 建站日期:8-24-2009 :::