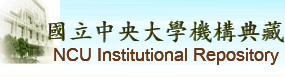 |
English
|
正體中文
|
简体中文
|
全文筆數/總筆數 : 81570/81570 (100%)
造訪人次 : 47015569
線上人數 : 174
|
|
|
資料載入中.....
|
請使用永久網址來引用或連結此文件:
http://ir.lib.ncu.edu.tw/handle/987654321/86846
|
題名: | 基於工廠生產資料的異常機器維修預測;Predictive maintenance of abnormal machines based on the factory production data |
作者: | 林聖洋;Lin, Sheng-Yang |
貢獻者: | 資訊工程學系 |
關鍵詞: | 維修預測;推薦系統;生產資料;時間序列;Predictive Maintenance;Recommendation system;production data;time series |
日期: | 2021-10-26 |
上傳時間: | 2021-12-07 13:19:43 (UTC+8) |
出版者: | 國立中央大學 |
摘要: | 在工廠生產模式中,異常的機器容易在生產時造成產品損壞,導致不良品數量上升, 嚴重者更可能停機導致整條生產線呆滯。普遍工廠都會安排定期維修保養,希望減少此情 況發生。在智慧製造 4.0 中,提出了對機器加裝感應器或是紀錄器協助生產,即便感應器 或紀錄器在生產中發出聲響提醒,此刻仍需停機維修,延誤了生產線。進而衍發出利用感 應器上的詳細資料進行剩餘維修剩餘天數預測。若機器能在異常前提早維修,便可減少不 良品以及省下不必要的停機時間,降低生產成本。然而並非每家工廠都願意額外花費安裝 感應器抑或者並非每部機器都可以嵌入安裝感應器來進行維修預測。 本研究針對上述問題,提出了一個利用工廠每週生產後提供的生產資料,建立了下 個週期的時間序列維修機器推薦列表模型。並使用了 Online Learning 的概念,隨著時間推 移更新每週的生產資料,預測下個週期的維修機器。利用實際生產維修紀錄當作比較結果, 維修預測列表中的機器在未來週期內皆會發生異常維修,可以利用來安排機器排程,避免 即將異常之機器進入產線。而考量工廠實際生產情況,使用六天(一周)的生產維修紀錄並 當為比較結果,十天的維修建議列表的平均 Recall 為 57.1%,再考量工廠每日維修能量有 限的情況下,將維修機器推薦列表進行排序,並給出前五名的名單十天的平均準確度為 58%,即建議清單中的五部機器有三部在未來六天內會發生維修。;In the factory production mode, the abnormal machines are easier damage the pieces during production, leading to increase the bad pieces’ number. What is more serious is the abnormal machines could stop that production line. All factories will arrange the regular maintenance, hoping to reduce the situation happened. In Smart Manufacturing 4.0, proposed a method to add sensors or recorders on the machines to assist in production. Even if the sensors or recorders makes a sound to reminder during production. It still needs to stop the production line to repair the machine, which delays the production line. After the concept of sensors, developing that using the detailed data on the sensors to predict the machines remaining useful life (RUL). If the machines can be repaired before abnormal conditions, it can reduce the bad pieces’ number and save the unnecessary stop production line time. The production cost can be reduced. However, not every factory is willing to spend extra money to install sensors or not every machine can embed with sensors for predictive maintenance. In response to the above problems, this research proposes a method. Using the production data provided by the factory every week to build a time series model of the recommended machine list. It also uses the concept of Online Learning to update weekly production data over time and predict the machines need to maintain in next period. Using the actual production maintenance record as the comparison result, all machines in recommended machine list will be abnormally repaired in the future period, the result can be used to arrange the machine schedule to prevent the abnormal machine will influence the production line. Considering the actual production situation of the factory, using the next six days (one week) maintenance as the comparison result, the average recall of ten days’ prediction of recommended machine list is 57.1%, and then considering the factory’s limited daily maintain energy, we decide a rule to rank the recommended machine list and only give the top 5 machine for recommended machine list. The ten days’ prediction of top 5 recommended machine list average precision is 58%, it means that there are three machines will be repaired in the recommended five machines. |
顯示於類別: | [資訊工程研究所] 博碩士論文
|
文件中的檔案:
檔案 |
描述 |
大小 | 格式 | 瀏覽次數 |
index.html | | 0Kb | HTML | 144 | 檢視/開啟 |
|
在NCUIR中所有的資料項目都受到原著作權保護.
|
::: Copyright National Central University. | 國立中央大學圖書館版權所有 | 收藏本站 | 設為首頁 | 最佳瀏覽畫面: 1024*768 | 建站日期:8-24-2009 :::