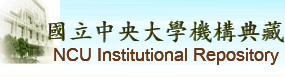 |
English
|
正體中文
|
简体中文
|
全文筆數/總筆數 : 81570/81570 (100%)
造訪人次 : 47010910
線上人數 : 288
|
|
|
資料載入中.....
|
請使用永久網址來引用或連結此文件:
http://ir.lib.ncu.edu.tw/handle/987654321/96289
|
題名: | 基於深度學習的樂器演奏動作識別;Action Recognition for Music Instruments Playing based on Deep Learning |
作者: | 赫巴特;Enkhbat, Avirmed |
貢獻者: | 資訊工程學系 |
關鍵詞: | 人類行為識別;影像分割;圖卷積網絡(GCN);時間卷積網絡(TCN);時空注意圖卷積網絡(STA-GCN);樂器;二胡;馬頭琴;Human action recognition;Image segmentation;Graph convolutional networks (GCN);Temporal convolutional networks (TCN);Spatial temporal attention graph convolutional network (STA-GCN);instrument;erhu;morin khuur |
日期: | 2024-11-11 |
上傳時間: | 2025-04-09 17:35:42 (UTC+8) |
出版者: | 國立中央大學 |
摘要: | 在人類行為識別(HAR)在樂器演奏中的應用,是一個重要的研究領域,通過利用人工智慧(AI)來提升音樂教育和演奏評估。這篇論文整合了兩個不同的研究方法:第一個方法著重於識別演奏二胡時的錯誤,第二個方法則探索馬頭琴的音符識別。這兩個方法都運用了深度學習技術,旨在開發能夠識別與樂器演奏相關的複雜動作和模式的模型。 二胡研究應用了圖卷積網絡(GCN)和時間卷積網絡(TCN),以捕捉人類骨骼運動的空間和時間關係。此研究的主要目標是檢測演奏中的錯誤,如手位不正、弓角不當及姿勢問題。系統通過分析演奏者的身體動作,識別影響演奏的技術錯誤,並提出改進技巧的建議。 另一方面,馬頭琴的演奏分析側重於音符識別,而非錯誤檢測。該研究使用了時空注意圖卷積網絡(STA-GCN),以捕捉手部關鍵點與樂器分割資訊之間的關係,從而識別正在演奏的音符。系統分析演奏者的連續手勢並將其映射到相應的音符。音符識別模型達到了81.4%的準確率,展示了其通過手勢識別來分析音樂作品的潛力。 為了支持這兩種方法,研究開發了全面的數據集,涵蓋了專業音樂家和初學者的二胡和馬頭琴演奏。這些數據集捕捉了音樂表演的細節,包括手部動作、指法位置、運弓技巧和音符轉換。針對每種樂器的獨特演奏技巧和表現動態,分別建立了單獨的數據集。 基於二胡的錯誤檢測系統顯示出高準確率,達到了97.6%,能夠識別演奏動作並找出手部和姿勢對齊的常見錯誤。相較之下,馬頭琴音符識別系統在通過手勢識別音符方面達到了81.4%的準確率。這兩個系統在各自領域中展現了潛力,突顯了結合深度學習模型與音樂表演分析的有效性。未來的研究將致力於擴展系統至更多樂器,並優化模型以提升識別與修正的表現。 本研究為音樂科技領域做出了貢獻,提供了針對傳統樂器演奏精度與音符識別的AI驅動解決方案。通過針對二胡和馬頭琴演奏需求量身定制的深度學習技術,本論文提供了一種新穎的人類行為識別與音樂分析方法。 ;Human action recognition (HAR) in musical instrument performance is an important research area that leverages artificial intelligence (AI) to enhance music education and performance evaluation. This thesis integrates two distinct research approaches: the first focuses on identifying errors while playing the erhu, and the second explores musical note recognition for the morin khuur. Both approaches utilize deep learning techniques to develop models capable of recognizing complex movements and patterns associated with musical instrument performance. The erhu study applies Graph Convolutional Networks (GCN) and Temporal Convolutional Networks (TCN) to capture both the spatial and temporal relationships of human skeletal movements. The primary objective of this research is to detect performance errors such as incorrect hand positioning, improper bow angles, and posture issues. The system analyzes the body movements of musicians, identifying technical errors that affect performance, and suggests ways to improve playing technique. On the other hand, the morin khuur performance analysis focuses on musical note recognition rather than error detection. This research involves the use of Spatial Temporal Attention Graph Convolutional Networks (STA-GCN) to capture the relationship between hand keypoints and instrument segmentation information in order to recognize the musical notes being played. The system analyzes the continuous gestures of musicians and maps them to the corresponding musical notes. The note recognition model achieved an accuracy of 81.4%, demonstrating its potential for analyzing musical compositions through gesture recognition. To support these two approaches, comprehensive datasets were developed, involving both professional musicians and beginners playing the erhu and morin khuur. These datasets capture the intricacies of musical performance, including hand movements, finger positioning, bowing techniques, and note transitions. Separate datasets were built for each instrument to handle their unique playing techniques and performance dynamics. The erhu-based error detection system demonstrated high accuracy, achieving 97.6% in recognizing playing actions and identifying common errors in hand and posture alignment. In contrast, the morin khuur note recognition system achieved an accuracy of 81.4% in recognizing musical notes from player gestures. Both systems showed potential in their respective areas, highlighting the effectiveness of combining deep learning models with musical performance analysis. Future work will focus on expanding the systems to additional instruments and optimizing the models to improve recognition and correction performance. This research contributes to the field of music technology by offering distinct AI-driven solutions for improving both performance accuracy and note recognition in traditional musical instruments. By applying deep learning techniques tailored to the specific needs of erhu and morin khuur performances, this thesis offers a novel approach to action recognition and musical analysis. |
顯示於類別: | [資訊工程研究所] 博碩士論文
|
文件中的檔案:
檔案 |
描述 |
大小 | 格式 | 瀏覽次數 |
index.html | | 0Kb | HTML | 16 | 檢視/開啟 |
|
在NCUIR中所有的資料項目都受到原著作權保護.
|
::: Copyright National Central University. | 國立中央大學圖書館版權所有 | 收藏本站 | 設為首頁 | 最佳瀏覽畫面: 1024*768 | 建站日期:8-24-2009 :::