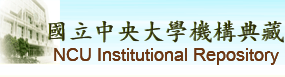 |
English
|
正體中文
|
简体中文
|
全文筆數/總筆數 : 80990/80990 (100%)
造訪人次 : 41625304
線上人數 : 1948
|
|
|
資料載入中.....
|
請使用永久網址來引用或連結此文件:
http://ir.lib.ncu.edu.tw/handle/987654321/89028
|
題名: | 使用 Himawari-8 AHI 觀測資料結合深度神經網絡估計大氣總可降水量;Estimation of Total Precipitable Water using Deep Neural Network Technique from Himawari-8 AHI Observation |
作者: | 劉佳怡;Liu, Chia-Yi |
貢獻者: | 大氣科學學系 |
關鍵詞: | 總可降水量 (TPW);深度神經學習 (DNN);Total Precipitable Water (TPW);Deep Neural Network (DNN) |
日期: | 2022-09-15 |
上傳時間: | 2022-10-04 10:48:22 (UTC+8) |
出版者: | 國立中央大學 |
摘要: | 水氣為大氣中重要的氣體之一,它可能影響降雨、劇烈天氣、水文循環和氣候變化等。然水氣具有在時空維度上快速變化的特點,因此,大範圍連續性的時間與空間觀測對於其變化的守視監測是非常重要的。因此利用地球同步氣象衛星所酬載之先進成像儀的發展,可穩定提供監測大氣環境的高時空解析度的資料。面對大量非線性的衛星觀測資料,為了在有效時間內進行水氣的估算,我們需要一種快速演算法來實現這一目標。因此本研究將嘗試以Himawari-8/Advanced Himawari Imager (AHI)資料結合深度神經網絡(DNN)構建TPW反演推估模型,並使用RAOB、SNPP-CrIS/ATMS、Passive Microwave Radiometer 和ground-based GNSS等觀測料確認其模型的可靠性。 研究的初步結果顯示,DNN-based H8/AHI估算的水氣與積分RAOB各層的水氣得到的TPW相比,具有良好的相關係數和較低的RMSE (分別為0.89 and 5.82 mm)。與SNPP-CrIS/ATMS L2 TPW資料相比,其0.846 的相關係數和RMSE 4.663 mm 也表明對於擁有微波觀測頻道所反演出的TPW有著一致性。在輸入變量因子之重要性的分析中,發現地表壓力、波長13.3μm的亮溫和緯度等資訊,對於TPW的推估具有較大的正面影響。此外,我們通過使用Passive Microwave Radiometer和ground-based GNSS觀測進行的分析中顯示,H8/AHI TPW的趨勢與Passive Microwave Radiometer在時序上的趨勢是相近平行的,並且與ground-based GNSS TPW相比具有不錯的相關係數 (0.656)。這顯示本研究所開發的DNN-based H8/AHI TPW反演推估模型,可具有在時序上連續反演的能力。;Water vapor is one of the most important constituents of the atmosphere. It might affect rainfall, severe weather, hydrological cycle and climate change. How-ever, water vapor has the characteristics that is changing rapidly in space-time dimension. Therefore, the monitoring of the change, we need the observation in large spatial and continuous in time domains. In recent years, with the advent of imaging instrument onboard geostationary satellites, which have ability to monitor atmos-pheric conditions with high spatiotemporal resolution, the high-quality full disk data have become available. In order to retrieve the total precipitable water (TPW) using the large number of nonlinear satellite data and be processed in an efficient time, we need a quick algo-rithm to achieve this goal. This study uses Himawari-8/Advanced Himawari Imager (AHI) data combined with Deep Neural Network (DNN) to build the TPW model, and uses the RAOB, SNPP-CrIS/ATMS, passive microwave radiometer and ground-based GNSS observation data to con-firm the reliability of this model. Pre-liminary results show the TPW which estimated by DNN-based H8/AHI have good correlation coefficients and low RMSE against TPW from integration of RAOB ob-servation in 0.89 and 5.82 mm, respectively. It also performs well in correlation co-efficient of 0.846 and RMSE of 4.663 mm when compared to SNPP-CrIS/ATMS data. In Variable Importance analysis, it is found that surface pressure, Tb16 (13.3μm) and latitude have larger positive impacts on the TPW estimation. Additional comparison had been conducted by using ground-based passive microwave radiometer and ground-based GNSS observation. The results show the tendency is in parallel in time with passive microwave radiometer and DNN-based H8/AHI has a higher correlation coefficient (0.656) compared with ground-based GNSS TPW. This suggests that DNN-based H8/AHI TPW model has the capability to retrieve TPW in time domain continuously. In conclusion, the developed DNN-based H8/AHI TPW model has good performance to estimate atmospheric water vapor information, and could pro-vide an alternative choice to supply timely information of precipitable water. |
顯示於類別: | [大氣物理研究所 ] 博碩士論文
|
文件中的檔案:
檔案 |
描述 |
大小 | 格式 | 瀏覽次數 |
index.html | | 0Kb | HTML | 89 | 檢視/開啟 |
|
在NCUIR中所有的資料項目都受到原著作權保護.
|
::: Copyright National Central University. | 國立中央大學圖書館版權所有 | 收藏本站 | 設為首頁 | 最佳瀏覽畫面: 1024*768 | 建站日期:8-24-2009 :::