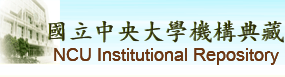 |
English
|
正體中文
|
简体中文
|
全文筆數/總筆數 : 80990/80990 (100%)
造訪人次 : 41638283
線上人數 : 1716
|
|
|
資料載入中.....
|
請使用永久網址來引用或連結此文件:
http://ir.lib.ncu.edu.tw/handle/987654321/93118
|
題名: | 應用文字探勘技術預測企業財務舞弊:以 PTT 股票版及重大訊息為例;Using Text Mining Techniques to Predict Financial Fraud: Taking PTT Stock and Material Information as an example |
作者: | 蘇郁雅;SU, YU-YA |
貢獻者: | 資訊管理學系在職專班 |
關鍵詞: | 舞弊預測;社群評論;重大訊息;文字表示方法;Fraud Prediction;Social Media Reviews;Material Information;Text Representations |
日期: | 2023-06-28 |
上傳時間: | 2024-09-19 16:43:16 (UTC+8) |
出版者: | 國立中央大學 |
摘要: | 職場舞弊行為不僅對企業財務及商譽造成損失,同時對投資者、員工等利害關係人及社會經濟產生負面影響。現行舞弊預測研究主要使用財務指標或財務報告進行預測,本研究係探討使用企業外部和內部及時文本資料,以社群評論和重大訊息公告為資料來源,並結合不同文字表示方法及分類模型進行實驗,評估可行性及預測效果。 本研究選取2012年至2022年4月於投資人保護中心之求償案件及台灣經濟新報資料庫中,發生舞弊事件之二十家公司,並以資產規模相近之二十家一般公司作為對照,收集新聞曝光前十八個月至新聞曝光前一日之PTT股票版留言及重大訊息主旨。本研究以PTT股票版留言、重大訊息主旨及結合前述二類為資料集,使用三種類型之文字表示方法及分類模型,分別為詞頻—逆向檔案頻率(Term Frequency-Inverse Document Frequency, TF-IDF) 搭配機器學習分類模型、Word2Vec詞向量搭配深度學習模型,以及中文預訓練語言模型BERT與RoBERTa分別建立舞弊偵測模型,並透過超參數優化方式提高模型性能,以比較不同資料集、文字表示方法和分類模型在預測效果之差異。 實驗結果顯示,使用中文RoBERTa語言模型進行微調後,達到最佳之預測效果。使用重大訊息主旨資料集,其AUC (Area Under Curve) 達0.91;使用PTT股票版留言及結合重大訊息主旨及PTT股票版留言資料集之AUC皆達0.82,顯示此三類資料集皆可有效預測舞弊。本研究提供內外部查核人員透過消息面觀點獲取舞弊風險之方法,同時可作為查核資源分配之參考。;Occupational fraud has detrimental impacts on companies, stakeholders, employees, and the economy, resulting in financial and reputational losses. Existing research on fraud prediction primarily relies on financial indicators or reports. In contrast, this study utilized real-time textual data from external and internal sources of enterprises, focusing on community comments and material information. The objective is to evaluate the feasibility and predictive performance of various text representation methods and classification models. This study collected data from 20 companies that experienced fraud incidents between 2012 and April 2022, identified from the Securities and Futures Investors Protection Center and the Taiwan Economic Journal (TEJ) database. For comparison, 20 non-fraudulent companies with similar asset scales were included. The dataset comprised text data from PTT stock reviews, material information headlines, and their combination within the 18 months prior to news exposure. 3 datasets utilized Term Frequency-Inverse Document Frequency (TF-IDF) with machine learning models, Word2Vec with deep learning models, and Chinese pre-trained language models (BERT and RoBERTa) to predict fraud. Hyperparameter optimization was performed to enhance model performance and prediction effects were compared across datasets, text representation methods, and classification models. The fine-tuned Chinese RoBERTa model achieved the best predictive performance with Area Under Curve (AUC) of 0.91 for material information headlines and 0.82 for PTT stock reviews and combined datasets, demonstrating effective fraud prediction across all 3 datasets. This study equips auditors with the ability to identify potential fraud risks from both internal and external perspectives, providing a resource for optimizing audit resource allocation. |
顯示於類別: | [資訊管理學系碩士在職專班 ] 博碩士論文
|
文件中的檔案:
檔案 |
描述 |
大小 | 格式 | 瀏覽次數 |
index.html | | 0Kb | HTML | 18 | 檢視/開啟 |
|
在NCUIR中所有的資料項目都受到原著作權保護.
|
::: Copyright National Central University. | 國立中央大學圖書館版權所有 | 收藏本站 | 設為首頁 | 最佳瀏覽畫面: 1024*768 | 建站日期:8-24-2009 :::