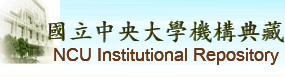 |
English
|
正體中文
|
简体中文
|
全文筆數/總筆數 : 80990/80990 (100%)
造訪人次 : 41266192
線上人數 : 110
|
|
|
資料載入中.....
|
請使用永久網址來引用或連結此文件:
http://ir.lib.ncu.edu.tw/handle/987654321/86909
|
題名: | 一個適用於量化深度神經網路且可調整精確度的處理單元設計: 一種階層式的設計方法;A Precision Reconfigurable Process Element Design for Quantized Deep Neural Networks: A Hierarchical Approach |
作者: | 徐麒惟;Hsu, Chi-Wei |
貢獻者: | 電機工程學系 |
關鍵詞: | 量化神經網路;運算單元;可重組式設計;Quantized Neural Networks (QNN);Processing Element (PE);Reconfigurable Design |
日期: | 2021-10-26 |
上傳時間: | 2021-12-07 13:25:08 (UTC+8) |
出版者: | 國立中央大學 |
摘要: | 卷積神經網路 (Convolution Neural Networks, CNN)在現今發展得十分迅速,主要使用在影像辨識、自駕車、物件偵測……等等。當我們應用CNN時,精準度以及資料大小是兩個重要的指標來計算效能以及運算效率。在傳統的CNN網路中,大部分都是以浮點數32bits來做計算以保持高水平的精準度。然而,要使用浮點數32bits運算必須用到32bits的乘加器 (MAC),這樣除了會在運算效率上造成瓶頸之外,還會使功耗大幅的上升,因此現今的研究者都在是利於找出減少資料量以此為加速的方法。量化(Quantization)是其中一種可以在精準度不下降太多的情況下來降低資料量已獲得加速的好處以及減少運算複雜度的一個方法。在CNN網路中,每次層所需要的位元數都不盡相同,而為了權衡更好的運算效率及精準度,不同的位元的運算會用在CNN網路的不同層數中,以增加運算效率。在以上的前提下,可以調整位元數的運算單元(Processing Element, PE)可以支援不同元位元的運算,像是 8bits x 8bits、 8bits x 4bits、4bits x 4bits以及2bits x 2bits。而我們所提出的這個架構屬於階層式的架構,這樣可以在設計過程中減少一些多餘的硬體,降低整體晶片的面積,而為了提升運算速度,我們提出的8bits x 8bits PE 可以做到兩級的平行化。而在實驗的部分,我們採用90nm的製程,從實驗結果中我們可以發現,跟先前的論文相比,我們2bits x 2bits面積可以減少57.5% - 68%,而在8bits x 8bits PE中,使用平行化架構可以讓8bits x 8bits的運算速度跟4bits x 4bits PE的運算速度相當。;In deep learning field, Convolution Neural Networks (CNNs) have been achieved a significant success in many fields such as visual imagery analysis, self-driving car, respectively. However, data size and the accuracy of each system are the major target to estimate the efficient and effective computations. In conventional CNN models, 32bits data are frequently used to maintain high accuracy. However, performing a bunch of 32bits multiply-and-accumulate (MAC) operations causes significant computing efforts as well as power consumptions. Therefore, recently researchers develop various methods to reduce data size and speed up calculations. Quantization is one of the techniques which reduces the number of bits of the data as well as the computational complexity at the cost of accuracy loss. To provide better computation effort and accuracy trade-off, different bit number may be applied to different layers within a CNN model. Therefore, a flexible processing element (PE) which can support operations of different bit numbers is in demand. In this work, we propose a hierarchy-based reconfigurable processing element (PE) structure that can support 8bits x 8bits, 8bits x 4bits, 4bits x 4bits and 2bits x 2bits operations. The structure we propose applies the concept of hierarchical structure that can avoid the redundant hardware in the design. To improve the calculation speed, our 8bits x 8bits PE applies two stage pipelines. The experimental results with 90nm technology show that in 2bits x 2bits PE, we can save the area by 57.5% to 60% compared to a Precision-Scalable accelerator. In the 8bits x 8bits PE, the two-stage pipelines can maintain almost the same calculation speed of the 4bits x 4 bits PE. |
顯示於類別: | [電機工程研究所] 博碩士論文
|
文件中的檔案:
檔案 |
描述 |
大小 | 格式 | 瀏覽次數 |
index.html | | 0Kb | HTML | 90 | 檢視/開啟 |
|
在NCUIR中所有的資料項目都受到原著作權保護.
|
::: Copyright National Central University. | 國立中央大學圖書館版權所有 | 收藏本站 | 設為首頁 | 最佳瀏覽畫面: 1024*768 | 建站日期:8-24-2009 :::