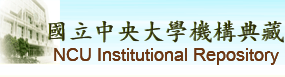 |
English
|
正體中文
|
简体中文
|
全文筆數/總筆數 : 80990/80990 (100%)
造訪人次 : 41077162
線上人數 : 1100
|
|
|
資料載入中.....
|
請使用永久網址來引用或連結此文件:
http://ir.lib.ncu.edu.tw/handle/987654321/95448
|
題名: | 整合深度學習與傳統分類器於財務新聞對高漲幅股價預測之研究;A Study on Integrating Deep Learning and Traditional Classifiers for Predicting High-Volatility Stock Prices Using Financial News |
作者: | 鄭心愉;Cheng, Hsin-Yu |
貢獻者: | 資訊管理學系 |
關鍵詞: | 股價預測;文字探勘;自然語言處理;機器學習;深度學習;類別不平衡;Stock Price Prediction;Text Mining;Natural Language Processing;Machine Learning;Deep Learning;Class Imbalance |
日期: | 2024-07-03 |
上傳時間: | 2024-10-09 16:51:54 (UTC+8) |
出版者: | 國立中央大學 |
摘要: | 在當今經濟體系之中,由於Covid-19疫情的衝擊,股市的波動性顯著增加,進而凸顯了準確預測股價動向的必要性。傳統的股價預測方法,例如技術指標與統計分析,已在迅速變化的市場環境下展現出其限制。本研究致力於提出最佳的股價預測模型組合,該組合融合深度學習技術與傳統機器學習方法,尤其著眼於高風險及高報酬股票的預測領域。考量到現有股價預測的文獻大多集中於中低風險領域,缺乏對高風險、高報酬的投資需求,本研究擴展了研究範圍,包含不同風險等級的股票預測,並著手探討表現最優的文字特徵與分類器組合。鑒於高風險股票的漲幅資料相對稀少,存在明顯的類別不平衡問題。本研究將透過對多種文字特徵提取方法和分類器模型,以及採樣方法深入分析與比較,旨在提供針對不同情境下或股票類型的最佳預測模型組合,以提高股價預測的準確性和適用性。 本研究結果顯示,在所有情境下並不存在一個特定的特徵萃取方式與分類器組合能持續展現最佳表現。實際上,每一種特定情況均有其對應的最佳組合,顯示在股價預測模型的建立中,應依據不同的市場狀況和資料特性選擇合適的特徵萃取方法和分類器算法。此外,另一項重要的研究發現是,當面對資料不平衡的問題時,採用過採樣和混合採樣技術對資料進行平衡處理,可以有效改善股價預測的性能。這顯示在處理金融時間序列資料時,適當的資料前處理策略是提升預測模型表現的關鍵因素。 ;In the current economic system, the impact of the Covid-19 pandemic has significantly increased the volatility of the stock market, thereby highlighting the necessity for accurate stock price prediction. Traditional methods of stock price forecasting, such as technical indicators and statistical analysis, have shown their limitations in a rapidly changing market environment. This research is dedicated to proposing the best combination of stock price prediction models, integrating deep learning techniques with traditional machine learning methods, particularly focusing on the prediction of high-risk and high-reward stocks. Considering that existing literature on stock price prediction primarily focuses on low to medium-risk areas, lacking in catering to the needs for high-risk, high-reward investments, this study expands its scope to include predictions across different risk levels and explores the most effective combinations of textual features and classifier models. Given the relatively sparse data on significant price movements of high-risk stocks, there is a clear issue of class imbalance. This research will conduct a thorough analysis and comparison of various text feature extraction methods and classifier models, as well as sampling techniques, aimed at providing the best model combination for different scenarios or types of stocks, to enhance the accuracy and applicability of stock price predictions.
The results of this study indicate that there is no single combination of feature extraction method and classifier that consistently shows the best performance in all scenarios. In fact, each specific situation has its optimal combination, demonstrating that in the establishment of stock price prediction models, it is essential to choose the appropriate feature extraction method and classifier algorithm based on different market conditions and data characteristics. Moreover, another significant finding is that addressing data imbalance issues through oversampling and hybrid sampling techniques can effectively improve the performance of stock price predictions. This reveals that proper data preprocessing strategies are crucial for enhancing the performance of prediction models when dealing with financial time series data. |
顯示於類別: | [資訊管理研究所] 博碩士論文
|
文件中的檔案:
檔案 |
描述 |
大小 | 格式 | 瀏覽次數 |
index.html | | 0Kb | HTML | 15 | 檢視/開啟 |
|
在NCUIR中所有的資料項目都受到原著作權保護.
|
::: Copyright National Central University. | 國立中央大學圖書館版權所有 | 收藏本站 | 設為首頁 | 最佳瀏覽畫面: 1024*768 | 建站日期:8-24-2009 :::